Title
The impact of architecturally qualified data in deep learning methods for the automatic generation of social housing layouts
Description
The objective of this work is to explore the impact of the data in the automatic generation of social housing layouts with different deep learning models. The design of Social Housing is a subfield of architecture that necessitates, from the architect, a high degree of precision in refining layouts to effectively address both the programmatic requirements and the aspiration to attain the utmost architectural quality to guarantee inhabitants comfort. This dual challenge stands to gain significantly from the incorporation of data-driven generative processes bolstered by machine learning techniques in general and deep learning ones in particular. The most widely used dataset for data-driven layout generation with deep learning methods is RPLAN, a generic floor plan dataset available online. Although it contains a significant amount of plans, it lacks any information about the architectural quality criteria used for the selection of the data. We conducted an initial analysis of the architectural consistency and key quality features of RPLAN. Upon finding several issues that raise doubts about its suitability for training social housing generation models, we proceeded to create PUBLICPLAN, a new dataset based on proposals submitted and evaluated in public architecture competitions in Spain over the past three years. It comprises 2446 distinct layouts extracted from 1279 proposals in Catalonia and the Balearic Islands. All of these layouts demonstrate high architectural standards. Then, we retrained the three most advanced existing models for generating interior plans using PUBLICPLAN and compared their performance to the same models trained with different subsets of RPLAN. Once the models were trained, we conducted several experiments comparing different models and data subsets and evaluating the impact of the quantity and quality of the data on the models’ performance. Those experiments involved over two hundred architects and architecture students. We also examined the plausibility of the generated plans being synthetic with a survey asking to distinguish between human and machine generated layout plans. Our results showed that PUBLICPLAN had a significant impact compared to the alternatives with a similar number of cases. In the examination of the feasibility of human-generated plans, over 48.43% of architects and architecture students identified machine-generated plans as being of human origin. Moreover, in qualitative experiments conducted with an equivalent dataset, our qualified dataset PUBLICPLAN demonstrated a significantly superior human rating (72.13%) when evaluated by human assessors, as compared to models trained with RPLAN. This highlights the importance of using qualified datasets in deep learning for the generation of social housing layouts. We also identified challenges and priorities in the research field, both in the deep learning process and architectural design practices.
Co-authors: Laura Carrera, Nil Brullet, Julia Capomaggi, Amadeu Santacana, Ricardo Devesa, Guillem Rosselló, Enrique Romero, Lluís Ortega.
Author: Ricardo Devesa
Editor: Erica Sogbe
Graphic Design: Fernando Guerrero
Details
Client: AAutomation in Construction. Volume 158
Date: 2024, February
More info
cover
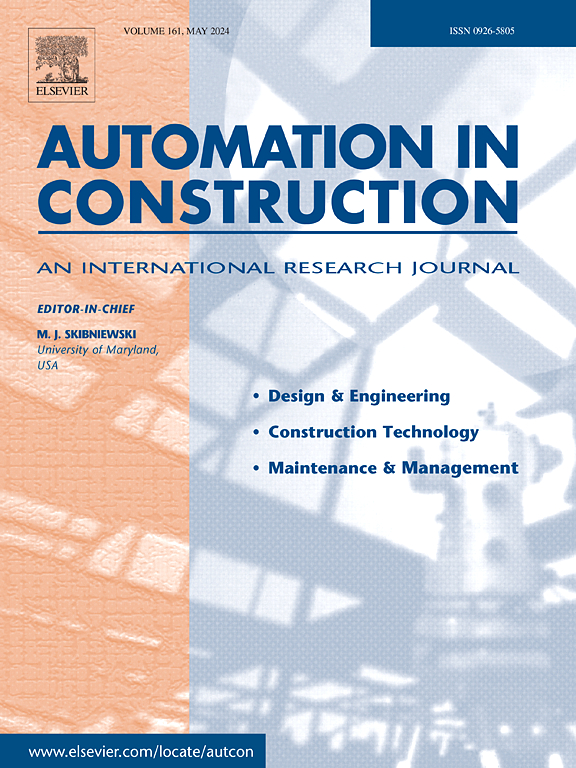